Authors: Giuseppe Modica, Joao Silva and Giandomenico De Luca.
The availability of very high resolution (VHR) images acquired from sensors assembled on unmanned aerial vehicles (UAVs) has introduced a new set of possibilities for classifying land cover types at finer levels of spatial detail, thus granting environmental monitoring with more precision and efficiency while opening up new perspectives in forest parameter evaluation and in the study of ecosystem functions. Geographical object-based image analysis (GEOBIA) is the most suitable classification method for this type of data, and it has replaced the pixel-based approach.
In our work, the main objective was to develop a supervised classification procedure of three vegetation layers (trees, shrubs and grass) in a Mediterranean cork oak woodland, located in Central Portugal, by applying a GEOBIA workflow, implemented in Orfeo Toolbox (OTB), to VHR imagery. It was operated through a second open-source software (QGIS) used as a graphical interface that enables a graphical analysis of data processing in real time. Data were acquired using a fixed-wing UAV with a modified RGB (Red Green Blue) camera (in which the blue channel was replaced by an NIR channel) and processed through photogrammetric and image processing procedures. Two orthomosaics were derived from two flights that were carried out in different and contrasting seasons: spring and summer. Successively, a window of the original scene was made so that only a smaller area of interest was classified (approximately 16.85 ha).
The implemented workflow has four main steps (Figure 1): preprocessing, segmentation, classification, and accuracy assessments. At the first, to normalize each band to a common range of values, a linear band stretching was performed (from 0 to 255). In order to increase the processing quality, we tested a methodology based on the combination of spectral and semantic information to improve the classification procedure through the combined use of three informative layers: R-G-NIR mosaics, NDVI (Normalized Difference Vegetation Index), and DSM (Digital Surface Model). Therefore, after a layer stacking process we obtained two final five-band orthomosaics, which were the input data for OTB. In order to measure the separability achieved by the original bands (R-G-NIR), NDVI and DSM for the different types of vegetation present in the study area (cork oak, shrubs, and grass) and, consequently, their importance for the classification, we implemented the modified M-statistic.
In OTB, the image object segmentation was performed by means of the Large-Scale Mean-Shift (LSMS) algorithm, and composed of four successive steps: (1) LSMS-smoothing; (2) LSMS-segmentation; (3) LSMS-merging; (4) LSMS-vectorization. To improve segmentation and obtaining the most satisfactory results, which significantly affects the subsequent classification phase, several tests were performed using different values of the range radius and minimum region size parameters.
Two supervised object-oriented classification algorithms were compared: support vector machine (SVM) and random forest (RF). For each of the five land cover classes (cork oak, shrubs, grass, bare soil, and shadows), training polygons were manually selected on the output vector file of the LSMS-Vectorization, by on-screen interpretation. The accuracy assessment was performed comparing a set of selected validation polygons (ground truth) with the predicted vegetation class, from which confusion matrices for RF and SVM algorithms were generated.. Finally, producer’s and user’s accuracy and the kappa coefficient (Khat) were calculated. Default values of the OTB parameters for training and classification processes gave optimal results: for both spring and summer imagery, the validation of the obtained results shows a very high accuracy level for both the SVM and RF classifiers, with kappa coefficient values ranging from 0.928 to 0.973 for RF and from 0.847 to 0.935 for SVM. Furthermore, the land cover class with the highest accuracy for both classifiers and for both flights was cork oak, which occupies the largest part of the study area. Both classifiers did not require particular computational resources: they took a few seconds (from 1 to 10 seconds, including the training phase but excluding the selection of trainers that we performed manually).
This paper investigates and confirms the reliability of free and open-source algorithms used in the geographical object-based image classification (GEOBIA) of (VHR) imagery surveyed by (UAVs) for forest monitoring. As far as we are aware, this study is the first that uses multispectral R-G-NIR images with DSM and NDVI for the classification of forest vegetation from UAV images using open-source software (OTB). The OTB suite is free and open-source software that is regularly updated and has proven to provide free processing tools with a steep learning curve coupled with robust results in forest ecosystems that have a complex vertical and horizontal structure. As our findings show, OTB can effectively process UAV imagery when implemented on low-power hardware. Moreover, the use of sensors based on standard R-G-NIR imagery, has the significant advantage of being a very affordable solution in both scientific and operational contexts. Mounting this type of sensors on a fixed-wing UAV enables the surveying of surfaces spread over hundreds of hectares in a short time and has thus proven to be a very cost-effective and reliable solution for forest monitoring. Therefore, the proposed workflow may be highly valuable in an operational context. An open question worth investigating relates to the application of our findings to other forest ecosystems, considering that we adopted this methodology in a structurally complex Mediterranean forest ecosystem such as cork oak woodlands, which are characterized by the presence of shrubs and herbs in the understory as well as tree shadows.
In the following link, the full paper can be downloaded https://doi.org/10.3390/rs11101238
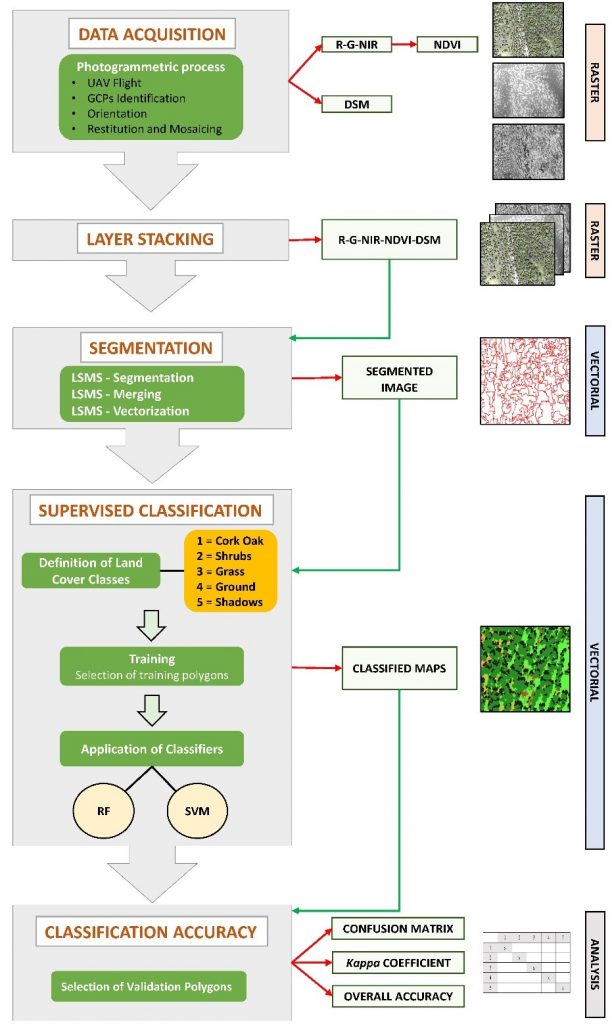
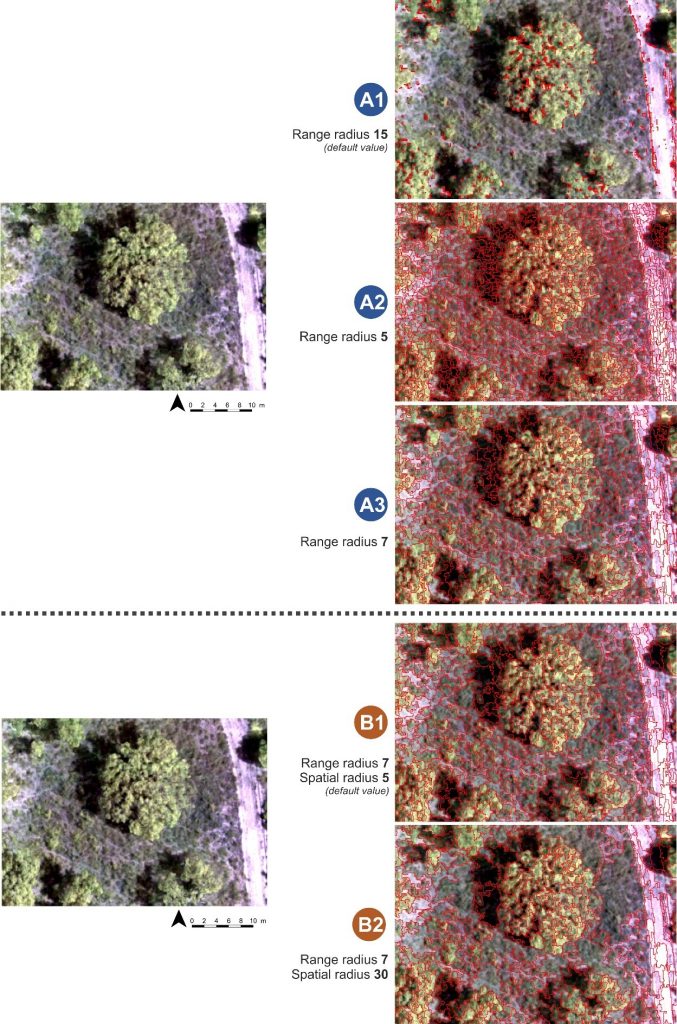